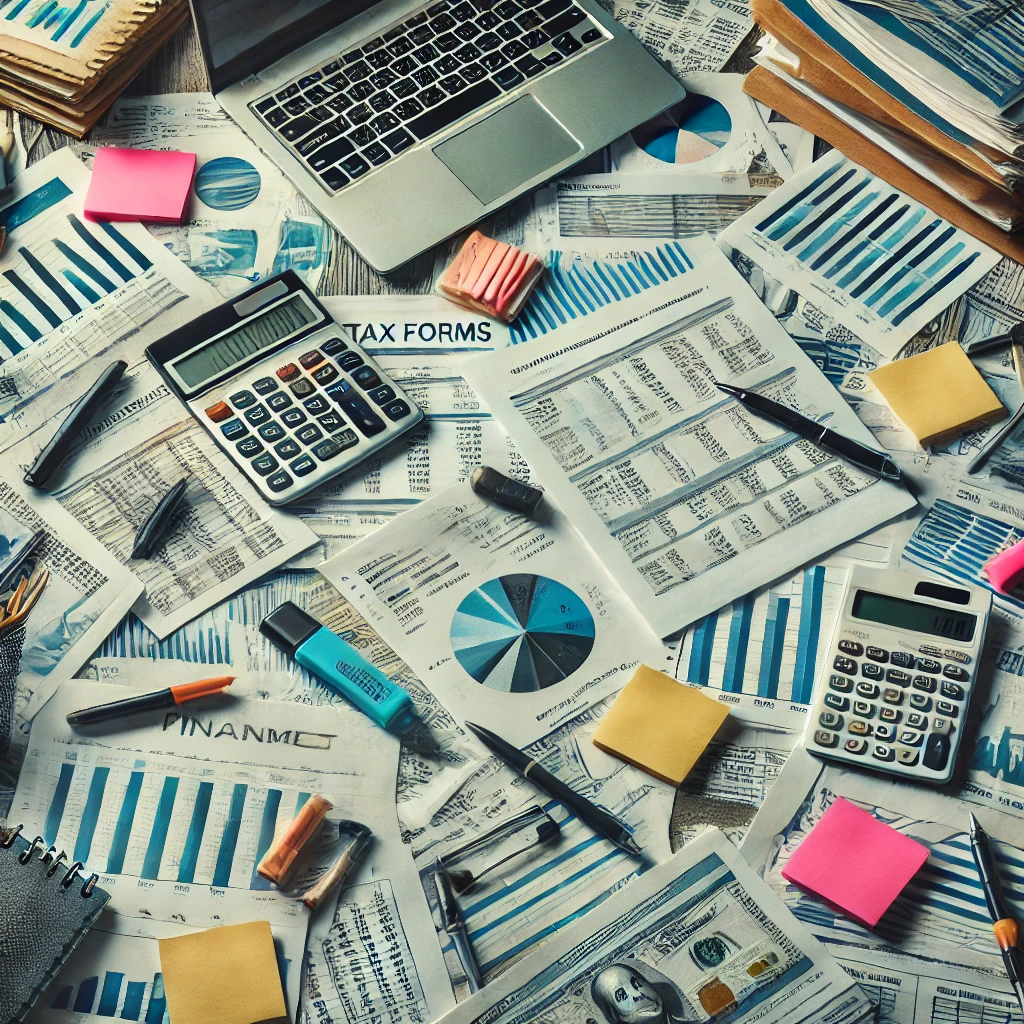
Background:
In today’s fast-paced financial technology landscape, startups and SMEs often face significant challenges in keeping up with the rapid evolution of AI-driven solutions. One such example is a small FinTech company based in Sydney, Australia, which was struggling to modernize its operations. The company, with a team of 15 employees, was heavily reliant on manual processes for customer transactions and compliance checks. This not only led to inefficiencies but also increased the risk of errors and non-compliance with regulatory standards.
The Founder and CTO, Michael Kim, was particularly concerned about the company’s ability to stay competitive. With limited resources to invest in AI talent and infrastructure, the company was at risk of falling behind both in terms of operational efficiency and regulatory compliance.
The Problem:
The challenges faced by this FinTech company are not unique. Many small and medium-sized enterprises (SMEs) in the financial sector struggle with:
- Operational inefficiencies: Manual processing of transactions and compliance checks is time-consuming and prone to human error. This leads to higher operational costs and slower service delivery.
- Customer experience: Without AI-driven personalization, it becomes difficult to offer tailored services that meet individual customer needs, which can negatively impact customer satisfaction.
- Regulatory compliance: The financial industry is heavily regulated, and staying compliant with evolving standards is a constant challenge. Manual compliance checks are not only inefficient but also increase the risk of non-compliance, which can result in hefty fines and reputational damage.
The Solution:
To address these challenges, the company needed a comprehensive AI-driven solution that could automate key processes, enhance customer experience, and ensure regulatory compliance. The approach involved several key components:
1. Data Integration:
The first step was to integrate the company’s existing data sources into a unified platform. This allowed for seamless data exchange and analysis, which is critical for any AI-driven solution. By consolidating data from various sources—such as transaction records, customer profiles, and compliance logs—the company could create a more holistic view of its operations.
2. AI-powered Transaction Processing:
The core of the solution was an AI engine, which we’ll refer to as FinGenie. This engine was trained on the company’s historical transaction data to automate the processing of customer transactions. By leveraging deep learning techniques, FinGenie was able to identify patterns in transaction data, detect anomalies, and flag suspicious activities in real-time. This not only reduced the time spent on manual transaction processing but also improved accuracy and fraud detection.
3. Regulatory Compliance:
To ensure adherence to regulatory requirements, a machine learning-based compliance framework was implemented. This framework continuously monitored transactions and other financial activities to ensure they met the necessary regulatory standards. By automating compliance checks, the company was able to achieve near-perfect accuracy in its compliance processes, significantly reducing the risk of non-compliance.
4. Talent Acquisition and Training:
While the AI-driven platform was a significant step forward, the company also needed to ensure that its team could effectively manage and maintain the new system. To address this, the company invested in upskilling its existing team through workshops and training sessions. This not only empowered the team to work with AI-driven tools but also fostered a culture of innovation within the organization.
Methodology:
The solution employed a range of AI-driven tools and techniques, including:
- Natural Language Processing (NLP): NLP was used for text analysis and sentiment analysis, particularly in customer interactions. This allowed the company to better understand customer feedback and tailor its services accordingly.
- Deep Learning: Deep learning models were used for pattern recognition and anomaly detection in transaction data. These models were able to identify subtle patterns that might indicate fraudulent activity or other irregularities.
- Predictive Analytics: Predictive models were employed for forecasting and risk assessment. By analyzing historical data, the company could predict future trends and make more informed decisions about risk management.
Results:
The implementation of this AI-driven platform led to several significant improvements:
- 40% reduction in operational costs: By automating transaction processing and compliance checks, the company was able to significantly reduce its operational costs. This allowed the team to focus on more strategic initiatives rather than getting bogged down in manual processes.
- 25% increase in customer satisfaction: The use of AI to personalize services and speed up transaction processing resulted in a noticeable improvement in customer satisfaction. Customers appreciated the faster service and the more tailored experience.
- 99.9% accuracy in compliance checks: The machine learning-based compliance framework ensured that the company remained fully compliant with regulatory requirements. This not only reduced the risk of fines but also enhanced the company’s reputation in the industry.
Actionable Insights and Recommendations:
For startups and SMEs facing similar challenges, there are several key takeaways from this solution:
- Prioritize Data Integration: A unified data platform is essential for any AI-driven solution. Without seamless data exchange, it becomes difficult to leverage AI effectively.
- Invest in AI Talent: While AI-driven platforms can automate many processes, having a team that understands how to manage and maintain these systems is crucial. Upskilling your existing team or bringing in external expertise can make a significant difference.
- Stay Up-to-Date with Regulatory Requirements: The financial industry is constantly evolving, and staying compliant with regulatory standards is a must. Regular audits and automated compliance checks can help ensure that your company remains on the right side of the law.
Conclusion:
The future of AI in financial technology is incredibly promising. As this example demonstrates, AI-driven solutions can have a transformative impact on operational efficiency, customer experience, and regulatory compliance. For startups and SMEs, the key is to start small, focus on data integration, and gradually build out AI capabilities that align with your business goals.
If you’re facing similar challenges or are curious about how AI can help your business, feel free to reach out for a friendly chat. We’re always happy to share insights and explore potential solutions tailored to your needs.
Have a Question? Let’s Chat!